Transforming Industries with Labelling Tool Machine Learning
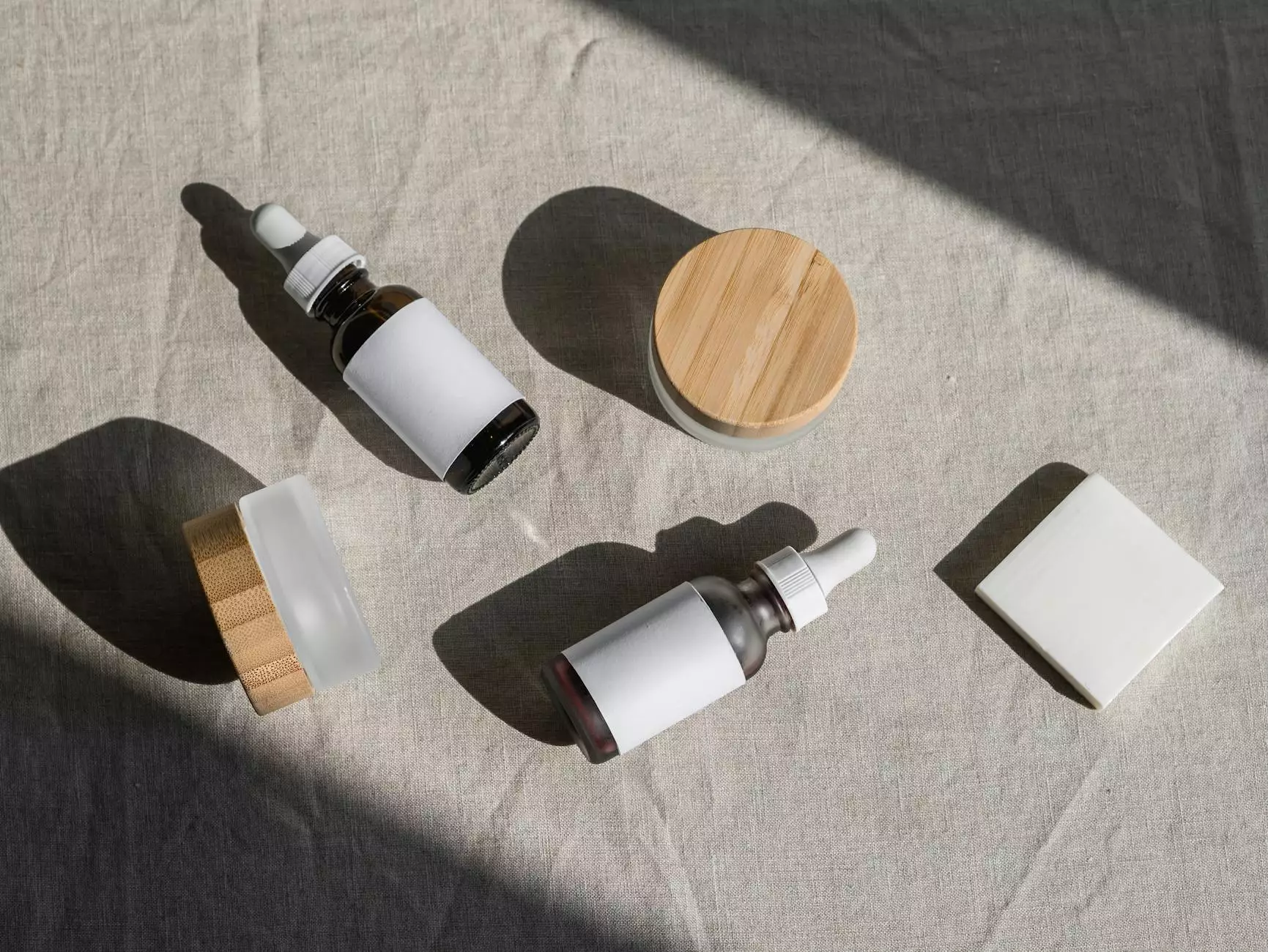
In the ever-evolving landscape of software development, one of the most transformative trends has been the rise of machine learning and its applications across various industries. Central to this transformation is the use of a labelling tool machine learning, which plays a crucial role in the preparation and enhancement of data. This article delves deep into how labelling tools integrated with machine learning are shaping the future of business processes, improving efficiency, and driving innovation.
Understanding the Importance of Data Labelling
Data is the bedrock of machine learning, but raw data alone is insufficient for creating effective models. Data labelling is the process of tagging, categorizing, and annotating data, making it understandable for machines. Inadequately labeled data can lead to poor model performance, making a robust labelling process pivotal. Here’s why data labelling matters:
- Model Accuracy: Well-labeled data leads to better model predictions and higher accuracy rates.
- Training Efficiency: Efficiently labeled datasets expedite the training process, reducing time and resources.
- Data Quality: Quality labels ensure that the machine learning model learns from precise and relevant data points.
The Role of Labelling Tools in Machine Learning
As businesses increasingly rely on data-driven decision-making, the demand for labelling tools has surged. These tools simplify and automate the data labelling process, thus significantly enhancing productivity. Here are some outstanding benefits of using labelling tools integrated with machine learning:
1. Enhanced Productivity
Labelling tools equipped with machine learning algorithms expedite the data annotation process. These tools can suggest labels based on patterns and previously labelled examples, significantly reducing the time that human annotators spend on the task. This productivity boost allows teams to focus on higher-level strategic tasks rather than tedious manual annotations.
2. Consistency and Accuracy
One of the challenges of manual labelling is inconsistency among different annotators. With automated labelling tools, organizations can ensure that labels are applied consistently across the dataset. This consistency is vital for training reliable machine learning models, as variations in labelling can lead to model bias and inaccuracies.
3. Cost Efficiency
Implementing labelling tool machine learning solutions can significantly cut down costs. By reducing the reliance on extensive human effort, businesses minimize labour costs while also decreasing the time to market for AI models. This cost-efficiency makes machine learning accessible to companies of all sizes, including startups with limited budgets.
4. Scalability
As the volume of data generated continues to accelerate, businesses require labelling solutions that can scale accordingly. Many modern labelling tools leverage cloud technologies or on-premise setups that easily scale to accommodate larger datasets without compromising performance.
Key Features of Modern Labelling Tool Machine Learning Solutions
Choosing the right labelling tool is crucial for harnessing the full potential of machine learning. Here are some of the key features to look for in a labelling tool:
- User-Friendly Interface: A simple and intuitive interface ensures that teams can quickly adapt and use the tool with minimal training.
- Integration Capabilities: The ability to easily integrate with existing workflows and systems is essential for seamless operations.
- Support for Various Data Types: Whether dealing with images, text, audio, or video, a robust labelling tool should accommodate diverse data types.
- Real-Time Collaboration: Features that enable multiple users to collaborate in real-time enhance productivity and speed up the labelling process.
Applications of Labelling Tool Machine Learning Across Industries
The applications of labelling tools in machine learning are extensive and varied across different sectors. Here are a few notable examples:
1. Healthcare
In healthcare, machine learning models are increasingly being employed for diagnostic assistance, predictive analysis, and patient management. Labelling tools are essential for annotating medical images, electronic health records, and genomic data to train models that can assist physicians more accurately and efficiently.
2. Autonomous Vehicles
The automotive industry is experiencing a revolution with the development of self-driving cars. Accurate labelling of road signs, pedestrian movements, and various environmental factors is crucial for training autonomous driving systems. Labelling tools help annotate video footage and sensor data, enabling manufacturers to build safer and more reliable vehicles.
3. Retail and E-commerce
In the retail sector, labelling tools assist in analyzing customer behavior, improving inventory management, and personalizing marketing efforts. By accurately labelling customer data and preferences, businesses can leverage machine learning to deliver tailored experiences, optimize pricing strategies, and enhance operational efficiency.
4. Financial Services
Financial institutions utilize machine learning for fraud detection, risk management, and customer service automation. Effective data labelling is necessary for training models that can identify fraudulent patterns, assess credit risks, and provide personalized financial advice.
Future Trends in Labelling Tool Machine Learning
As technology advances, the landscape of labelling tools continues to evolve. Here are some future trends to consider:
- Self-Learning Systems: Future labelling tools are likely to incorporate self-learning systems that improve their accuracy over time by learning from user corrections and feedback.
- AI-Powered Automation: Increased automation will streamline workflows, enabling businesses to fully harness the speed and efficiency of machine learning without overburdening their teams.
- Enhanced Augmented Reality: In sectors like retail and healthcare, AR technology will play a role in visualizing data labels and enhancing interactive experiences.
- Improved Collaboration Tools: Expect to see more features that facilitate collaboration among annotation teams, allowing for real-time updates and communication.
Choosing the Right Labelling Tool Machine Learning Solution
With numerous labelling tools available in the market, selecting the right one can be challenging. Here are critical steps to ensure you choose the best tool for your needs:
1. Define Your Requirements
Assess your project’s specific data types, volume, and complexity. Knowing what you need will guide you in selecting a tool that matches those requirements.
2. Evaluate User Experience
Prioritize tools with an intuitive interface that allows your team to use it effectively with minimal training. A good user experience often leads to higher productivity and better results.
3. Consider Integration
Your chosen tool should seamlessly integrate into your existing systems. Look for APIs and documentation that support easy integration.
4. Review Vendor Reputation
Research the vendor’s track record, customer support, and community feedback. This due diligence can save you time and resources down the road.
Conclusion
The integration of labelling tool machine learning in various sectors exemplifies how data can be transformed into actionable insights. By improving the accuracy and efficiency of data annotation, businesses can harness machine learning to drive innovation, optimize operational efficiency, and accelerate decision-making processes.
As this technology continues to evolve, organizations that adopt advanced labelling tools will be better positioned to leverage data effectively, ensuring they stay competitive in a data-driven marketplace. Understanding the potential impacts and navigating the available tools will fortify your business's journey into the future of machine learning.